前言
本文主要推荐一些生成对抗网络(GAN,generative adversarial networks)相关的值得精读的论文,主要涉及GAN的优化,图像翻译,视频预测三个领域,可以作为GAN的学习顺序进行阅读。
GAN的优化
想要使用GAN完成期望的学习任务,精致的网络设计和合适的目标函数必不可少,二者是实现较高performance的关键因素。
在损失函数的优化论文方面,效果比较突出且使用较多的主要有三篇论文,分别是:lsgan, wgan-gp, 谱归一化GAN:
- Improved training of Wasserstein GANs :https://arxiv.org/abs/1704.00028
- Least squares generative adversarial networks. : https://arxiv.org/abs/1611.04076
- Spectral normalization for generative adversarial networks:https://arxiv.org/abs/1802.05957
这三篇论文提出的loss或正则方法都对GAN的优化有不小的作用,笔者也都做过相关实验,效果突出。
除了损失函数,网络结构的设计也是完成GAN训练任务里重要的一部分,此方面可以参考的文章主要有以下几篇:
- Deep generative image models using a Laplacian pyramid of adversarial networks: https://arxiv.org/abs/1506.05751
特征金字塔GAN,(如下图)不同scale的图像可以提供不同精细程度的信息。
image.png-120.5kB
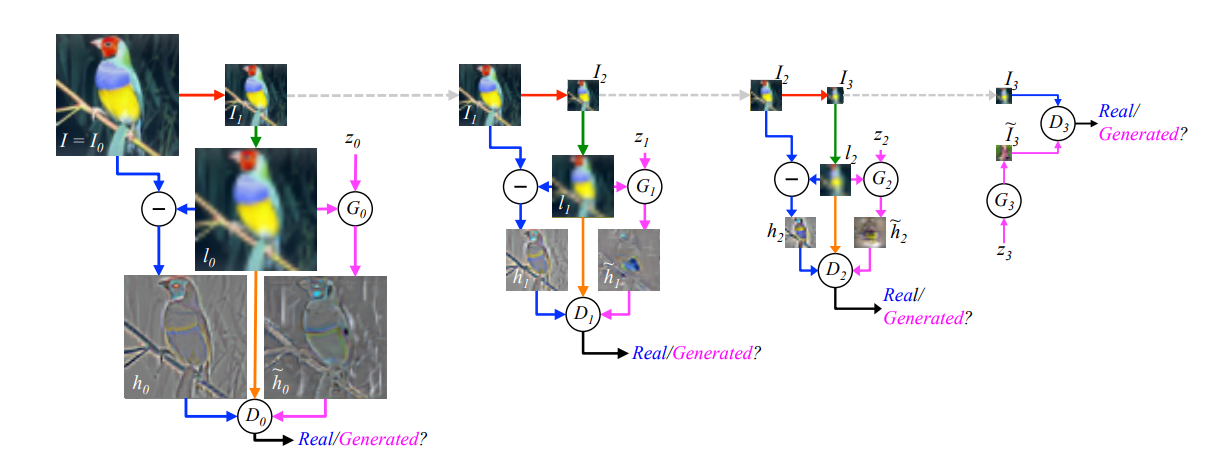
- Unsupervised representation learning with deep convolutional generative adversarial networks: https://arxiv.org/abs/1511.06434
这篇是DCGAN,文章主要就是推行卷积结构的GAN网络,包括采样层的设计,激活函数的选择等等。
3.Stacked generative adversarial networks :https://arxiv.org/abs/1612.04357
4.StackGAN: Text to photo-realistic image synthesis with stacked generative adversarial networks. : https://arxiv.org/abs/1612.03242
这两篇篇论文引出stackGAN, 表明生成器的串联在某些任务上可以实现更好的效果
-
Progressive growing of GANs for improved quality, stability, and variation: https://arxiv.org/abs/1710.10196
这篇是proGAN, 提出从最低分辨率的图像开始生成,逐渐生成更高分辨率的图像,在此过程中,网络结构由简单逐渐变得复杂。网络完成训练后,足以生成2K分辨率真假难辨的图像。 -
High-resolution image synthesis and semantic manipulation with conditional GANs:https://arxiv.org/abs/1711.11585
这篇论文在loss和网络结构上都有一些改进,很适合图像翻译任务。
图像翻译
图像翻译任务是指将图像从一个域转到另一个域,比如:风格迁移,图像上色,图像转换等等,代表作主要是pix-2-pix系列。这里有个图像翻译小demo,感兴趣可以试玩下:https://affinelayer.com/pixsrv/
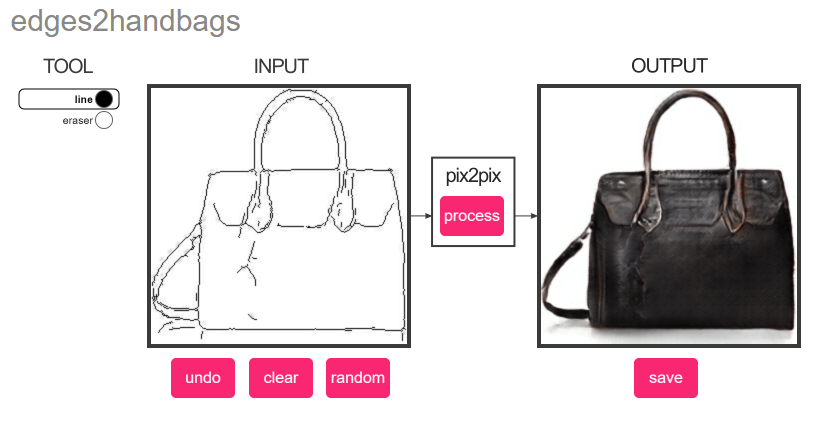
- Unsupervised pixel-level domain adaptation with generative adversarial networks :https://arxiv.org/abs/1612.05424
- Multimodal unsupervised image-to-image translation. : https://arxiv.org/abs/1804.04732
- Image-to-image translation with conditional adversarial networks. :https://arxiv.org/abs/1611.07004
- Unsupervised image-to-image translation networks. : https://arxiv.org/abs/1703.00848
- Coupled generative adversarial networks : https://arxiv.org/abs/1606.07536
- Learning from simulated and unsupervised images through adversarial training : https://arxiv.org/abs/1612.07828
- Unsupervised cross-domain image generation. : https://arxiv.org/abs/1611.02200
- High-resolution image synthesis and semantic manipulation with conditional GANs. : https://arxiv.org/abs/1711.11585
- Unpaired image-to-image translation using cycle-consistent adversarial networks. : https://arxiv.org/abs/1703.10593
- Toward multimodal image-to-image translation. : https://arxiv.org/abs/1711.11586
视频预测
GAN在图像上取得巨大成功的原因就是GAN可以很好学习到图像数据的数据分布并模拟出来,科研人员就开始将其应用到视频预测领域,根据视频的前一段时间发生的情况预测接下来的视频走向。这部分内容对研究视频合成,视频预测,异常检测等都有重要价值,也可以用来做一些有意思的demo。
- Unsupervised learning of disentangled representations from video : https://arxiv.org/abs/1705.10915
- Unsupervised learning for physical interaction through video prediction. : https://arxiv.org/abs/1605.07157
- Video pixel networks. : https://arxiv.org/abs/1610.00527
- Stochastic adversarial video prediction. : https://arxiv.org/abs/1804.01523
- Dual motion GAN for future-flow embedded video prediction. : https://arxiv.org/abs/1708.00284.
- Deep predictive coding networks for video prediction and unsupervised. : https://arxiv.org/abs/1605.08104
- Deep multi-scale video prediction beyond mean square error. : https://arxiv.org/abs/1511.05440
- Unsupervised learning of video representations using lstms. : https://arxiv.org/abs/1502.04681
- Decomposing motion and content for natural video sequence prediction. : https://arxiv.org/abs/1706.08033
- Generating the future with adversarial transformers. : https://ieeexplore.ieee.org/document/8099802
- The pose knows: Video forecasting by generating pose futures. : https://arxiv.org/abs/1705.00053
总结
本文主要推荐了一些GAN相关的文章,从理论分析到场景应用,从图像到视频。论文的推荐顺序既可以用来快速查找,也可以用来作为进阶的阅读顺序,人力有时穷,还有更多未提到的较好工作,欢迎补充。
BTW
从论文的链接上发现,大佬们的优秀成果都喜欢放在arxiv上,所以,大家希望探究最新的工作时,不妨直接去arxiv网站上找,这里给出链接:http://cn.arxiv.org/ 输入关键字,就可以查找希望看到的论文。
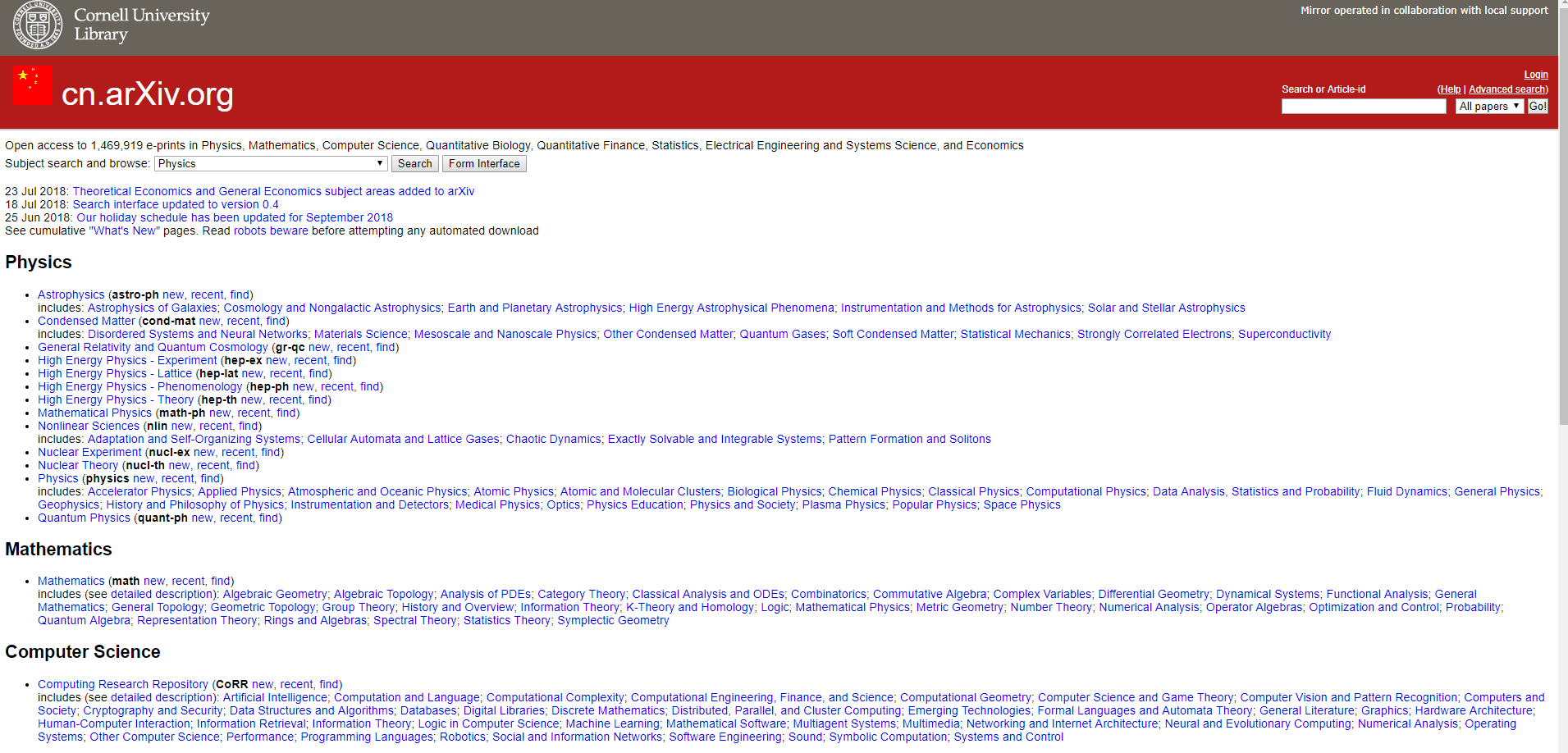
网友评论