Authors
Tuesday, 3 January 2017

Shane LeggCo-Founder & Chief Scientist, DeepMind
In a world of fiercely complex, emergent, and hard-to-master systems - from our climate to the diseases we strive to conquer - we believe that intelligent programs will help unearth new scientific knowledge that we can use for social benefit. To achieve this, we believe we’ll need general-purpose learning systems that are capable of developing their own understanding of a problem from scratch, and of using this to identify patterns and breakthroughs that we might otherwise miss. This is the focus of our long-term research mission at DeepMind.
While we remain a long way from anything that approximates what you or we would term intelligence, 2016 was a big year in which we made exciting progress on a number of the core underlying challenges, and saw the first glimpses of the potential for positive real-world impact.
Our program AlphaGo, for which we were lucky enough to receive our second Nature front cover, took on and beat the world champion Lee Sedol at the ancient game of Go, a feat that many experts said came a decade ahead of its time. Most exciting for us - as well as for the worldwide Go community - were AlphaGo’s displays of game-winning creativity, in some cases finding moves that challenged millennia of Go wisdom. In its ability to identify and share new insights about one of the most contemplated games of all time, AlphaGo offers a promising sign of the value AI may one day provide, and we're looking forward to playing more games in 2017.
We also made meaningful progress in the field of generative models, building programs able to imagine new constructs and scenarios for themselves. Following our PixelCNN paper on image generation, our paper on WaveNet demonstrated the usefulness of generative audio, achieving the world’s most life-like speech synthesis by imaginatively creating raw waveforms rather than stitching together samples of recorded language. We’re planning to put this into production with Google and are excited about enabling improvements to products used by millions of people.
Another important area of research is memory, and specifically the challenge of combining the decision-making aptitude of neural networks with the ability to store and reason about complex, structured data. Our work on Differentiable Neural Computers, for which we received our third Nature paper in eighteen months, demonstrated models that can simultaneously learn like neural networks as well as memorise data like computers. These models are already able to learn how to answer questions about data structures from family trees to tube maps, and bring us closer to the goal of using AI for scientific discovery in complex datasets.
As well as pushing the boundaries of what these systems can do, we’ve also invested significant time in improving how they learn. A paper titled ‘Reinforcement Learning with Unsupervised Auxiliary Tasks’ described methods to improve the speed of learning for certain tasks by an order of magnitude. And given the importance of high-quality training environments for agents, we open sourced our flagship DeepMind Lab research environment for the community, and are working with Blizzard to develop AI-ready training environments for StarCraft II as well.
Of course, this is just the tip of the iceberg, and you can read much more about our work in the many papers we published this year in top-tier journals from Neuron to PNAS and at major machine learning conferences from ICLR to NIPS. It’s amazing to see how others in the community are already actively implementing and building on the work in these papers - just look at the remarkable renaissance of Go-playing computer programs in the latter part of 2016! - and to witness the broader fields of AI and machine learning go from strength to strength.
It’s equally amazing to see the first early signs of real-world impact from this work. Our partnership with Google’s data centre team used AlphaGo-like techniques to discover creative new methods of managing cooling, leading to a remarkable 15% improvement in the buildings’ energy efficiency. If it proves possible to scale these kinds of techniques up to other large-scale industrial systems, there's real potential for significant global environmental and cost benefits. This is just one example of the work we’re doing with various teams at Google to apply our cutting-edge research to products and infrastructure used across the world. We’re also actively engaged in machine learning research partnerships with two NHS hospital groups in the UK, our home, to explore how our techniques could enable more efficient diagnosis and treatment of conditions that affect millions worldwide, as well as working with two further hospital groups on mobile apps and foundational infrastructure to enable improved care on the clinical frontlines.
Of course, the positive social impact of technology isn’t only about the real-world problems we seek to solve, but also about the way in which algorithms and models are designed, trained and deployed in general. We’re proud to have been involved in founding the Partnership on AI, which will bring together leading research labs with non-profits, civil society groups and academics to develop best practices in areas such as algorithmic transparency and safety. By fostering a diversity of experience and insight, we hope that we can help address some of these challenges and find ways to put social purpose at the heart of the AI community across the world.
We’re still a young company early in our mission, but if in 2017 we can make further simultaneous progress on these three fronts - algorithmic breakthroughs, social impact, and ethical best practice - then we'll be in good shape to make a meaningful continued contribution to the scientific community and to the world beyond.
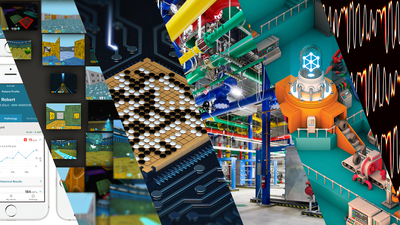
网友评论