本节笔记对应第三周Coursera课程 binary classification problem 更多见:李飞阳
Classification is not actually a linear function.
Classification and Representation
Hypothesis Representation
- Sigmoid Function(or we called Logistic Function)
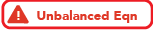 = g ( \theta^T x ) \newline \newline& z = \theta^T x \newline& g(z) = \dfrac{1}{1 + e^{-z}}\end{align})
Sigmoid Function 可以使输出值范围在$(0,1)$之间。$g(z)$对应的图为:

Solving the Problem of Overfitting
The Problem of Overfitting

address the issue of overfitting
- Reduce the number of features:
- Manually select which features to keep.
- Use a model selection algorithm (studied later in the course).
- Regularization:
- Keep all the features, but reduce the magnitude of parameters $θ_j$.
- Regularization works well when we have a lot of slightly useful features.
Cost Function

- in a single summation
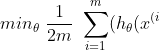}) - y{(i)})2 + \lambda\ \sum_{j=1}^n \theta_j^2)
The λ, or lambda, is the regularization parameter. It determines how much the costs of our theta parameters are inflated.
Regularized Linear Regression
- Gradient Descent
$$\begin{align} & \text{Repeat}\ \lbrace \newline & \ \ \ \ \theta_0 := \theta_0 - \alpha\ \frac{1}{m}\ \sum_{i=1}^m (h_\theta(x^{(i)}) - y{(i)})x_0{(i)} \newline & \ \ \ \ \theta_j := \theta_j - \alpha\ \left[ \left( \frac{1}{m}\ \sum_{i=1}^m (h_\theta(x^{(i)}) - y{(i)})x_j{(i)} \right) + \frac{\lambda}{m}\theta_j \right] &\ \ \ \ \ \ \ \ \ \ j \in \lbrace 1,2...n\rbrace\newline & \rbrace \end{align}$$
-
Normal Equation
$$\begin{align}& \theta = \left( X^TX + \lambda \cdot L \right)^{-1} X^Ty \newline& \text{where}\ \ L = \begin{bmatrix} 0 & & & & \newline & 1 & & & \newline & & 1 & & \newline & & & \ddots & \newline & & & & 1 \newline\end{bmatrix}\end{align}$$ -
L is a matrix with 0 at the top left and 1's down the diagonal, with 0's everywhere else. It should have dimension (n+1)×(n+1)
-
Recall that if m ≤ n, then $X^TX$ is non-invertible. However, when we add the term λ⋅L, then $X^TX + λ⋅L $becomes invertible.
Summary
我在这里整理一下上述两个方法,补全课程上的相关推导。
Logistic Regression Model
$h_\theta(x)$是假设函数
$$h_\theta (x) = g ( \theta^T x ) = \dfrac{1}{1 + e^{- \theta^T x}} $$
注意假设函数和真实数据之间的区别
Cost Function
$$J(\theta) = - \frac{1}{m} \sum_{i=1}^m \large[ y^{(i)}\ \log (h_\theta (x^{(i)})) + (1 - y^{(i)})\ \log (1 - h_\theta(x^{(i)}))\large]$$
回头看看上边的那个$h_\theta (x)$ ,cost function定义了训练集给出的结果 和 当前计算结果之间的差距。当然,该差距越小越好,那么需要求导一下。
Gradient Descent
- 原始公式
$$\theta_j := \theta_j - \alpha \dfrac{\partial}{\partial \theta_j}J(\theta)$$ - 求导计算
$$\frac{\partial}{\partial \theta_j} J(\theta) = \frac{1}{m}\sum\limits_{i=1}^{m}[(h_\theta (x^{(i)}) - y{(i)})x_j{(i)}]$$ - 计算结果
$$\theta_j := \theta_j - \frac{\alpha}{m} \sum_{i=1}^m (h_\theta(x^{(i)}) - y^{(i)}) x_j^{(i)} $$
这里推导一下$\frac{\partial}{\partial \theta_j} J(\theta)$:
-
计算$h_\theta'(x)$导数
$$\begin{align} &h_\theta'(x) = ( \frac1{1+e^{- \theta x}})'\newline &\ \ \ \ \ \ \ \ = \frac{e^{- \theta x}x}{1+e^{- \theta x}}\newline &\ \ \ \ \ \ \ \ = \frac{1+e^{- \theta x}-1}{(1+e^{- \theta x})^2}x\newline &\ \ \ \ \ \ \ \ = \large[\frac{1}{1+e^{- \theta x}}-\frac{1}{(1+e^{- \theta x})^2}\large]x\newline &\ \ \ \ \ \ \ \ = h_\theta(x)(1-h_\theta(x))x \end{align}$$ -
推导$\frac{\partial}{\partial \theta_j} J(\theta)$
$$\begin{align} &\frac{\partial}{\partial \theta_j} J(\theta) = \frac{\partial}{\partial \theta_j} \frac{1}{m} \sum_{i=1}^m \large[ -y^{(i)}\ \log (h_\theta (x^{(i)})) - (1 - y^{(i)})\ \log (1 - h_\theta(x^{(i)}))\large] \newline &\ \ \ \ \ \ \ \ \ \ \ \ \ \ = \frac{1}{m} \sum_{i=1}^m \large[ -y{(i)} \frac1{h_\theta(x{(i)})}h_\theta'(x^{(i)}) - (1 - y^{(i)}) \frac{-1}{1-h_\theta(x{(i)})}h_\theta'(x{(i)})\large] \newline &\ \ \ \ \ \ \ \ \ \ \ \ \ \ = \frac{1}{m} \sum_{i=1}^m \large[ -y{(i)} \frac1{h_\theta(x{(i)})}h_\theta(x{(i)})(1-h_\theta(x{(i)}))x^{(i)} \newline &\ \ \ \ \ \ \ \ \ \ \ \ \ \ \ \ \ \ \ \ \ \ \ \ \ \ \ \ \ \ \ \ \ \ \ \ \ \ \ \ \ \ \ \ \ \ \ \ \ \ \ - (1 - y^{(i)}) \frac{-1}{1-h_\theta(x{(i)})}h_\theta(x{(i)})(1-h_\theta(x{(i)}))x{(i)}\large] \newline &\ \ \ \ \ \ \ \ \ \ \ \ \ \ = \frac{1}{m} \sum_{i=1}^m \large[ -y{(i)}(1-h_\theta(x{(i)}) x^{(i)})+(1- y)h_\theta(x^{(i)}) x^{(i)})\large] \newline &\ \ \ \ \ \ \ \ \ \ \ \ \ \ = \frac{1}{m} \sum_{i=1}^m \large[ -x{(i)}y{(i)}+x{(i)}y{(i)}h_\theta(x^{(i)}) \newline &\ \ \ \ \ \ \ \ \ \ \ \ \ \ \ \ \ \ \ \ \ \ \ \ \ \ \ \ \ \ \ \ \ \ \ \ \ \ \ \ \ \ \ \ \ \ \ \ \ \ \ \ \ \ \ \ \ \ \ \ \ \ \ \ \ \ \ \ \ \ \ \ \ \ \ \ \ \ \ \ \ \ \ \ +x{(i)}h_\theta(x{(i)}) - x{(i)}y{(i)}h_\theta(x^{(i)}) \large] \newline &\ \ \ \ \ \ \ \ \ \ \ \ \ \ = \frac{1}{m}\sum\limits_{i=1}^{m}[(h_\theta (x^{(i)}) - y{(i)})x_j{(i)}] \end{align}$$
即:
$$\begin{align} &\frac{\partial}{\partial \theta_j} J(\theta) = \frac{1}{m}\sum\limits_{i=1}^{m}[(h_\theta (x^{(i)}) - y{(i)})x_j{(i)}] \end{align}$$
Solving the Problem of Overfitting
其他地方都一样,稍作修改
-
Cost Function
$$J(\theta) = - \frac{1}{m} \sum_{i=1}^m \large[ y^{(i)}\ \log (h_\theta (x^{(i)})) + (1 - y^{(i)})\ \log (1 - h_\theta(x^{(i)}))\large] + \frac{\lambda}{2m}\sum_{j=1}^n \theta_j^2$$ -
Gradient Descent
$$\begin{align} & \text{Repeat}\ \lbrace \newline & \ \ \ \ \theta_0 := \theta_0 - \alpha\ \frac{1}{m}\ \sum_{i=1}^m (h_\theta(x^{(i)}) - y{(i)})x_0{(i)} \newline & \ \ \ \ \theta_j := \theta_j - \alpha\ \left[ \left( \frac{1}{m}\ \sum_{i=1}^m (h_\theta(x^{(i)}) - y{(i)})x_j{(i)} \right) + \frac{\lambda}{m}\theta_j \right] &\ \ \ \ \ \ \ \ \ \ j \in \lbrace 1,2...n\rbrace\newline & \rbrace \end{align}$$
以上
网友评论